The highs, lows and future of ethical machine learning
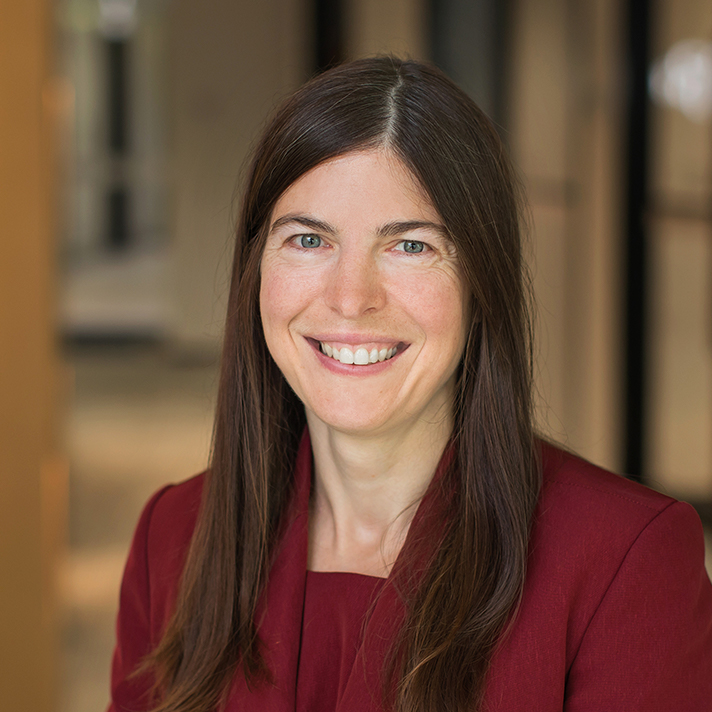
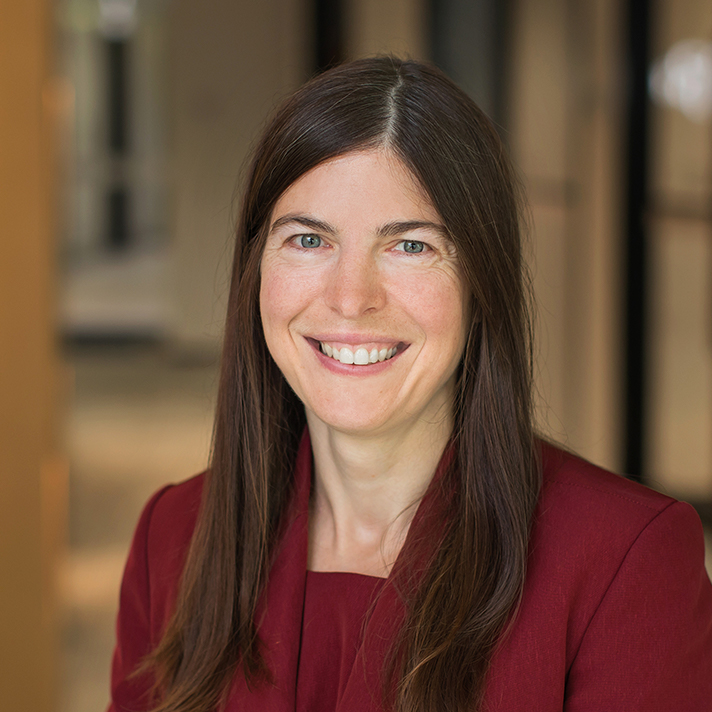
The world’s most valuable resource is no longer oil, but data - The Economist
Big data is the new oil. Just as oil enabled us to progress our civilisation, big data provides many opportunities for advancements and insights, primarily through machine learning and artificial intelligence. In the first of a two-part series, we explore the ups and downs of a booming industry and why ethics are increasingly centre stage.
What is machine learning?
Machine Learning typically refers to algorithms and statistical models that enable computers to draw inferences from patterns in data without having each step explicitly encoded by a human operator. Some of these algorithms may also self-adapt over time as new data is presented to the system. These systems may then be applied to tasks normally perceived to require human intelligence, such as visual perception, speech recognition, decision-making, and translation between languages.
Typically, machine learning requires large amounts of data to achieve accurate results. Developments in computing power, storage and the increased digitalisation of the world have created the conditions to facilitate this requirement and allow for the data-based revolution to take place.
What are the benefits of machine learning?
Machine learning delivers many opportunities …
Insights
It can help us find new relationships in data. For example:
- Finding similarities in bacterial and viral DNA sequences can help determine the evolutionary relationships between different strains. For disease-causing pathogens, this is an important tool for tracking outbreaks and to develop treatments.
- In the same general field, scientists believe a recent development could transform computational biology. DeepMind’s AlphaFold, which uses deep learning, has vastly improved the ability of computers to accurately predict the 3D shape of proteins, comprehensively outperforming the prior state-of-the-art algorithms in a recent global competition. Knowing the shape of proteins is important in many research areas, including genetic diseases and drug discovery.
Efficiency
It can lead to greater efficiencies in processes. Natural Language Processing (NLP) was used to mine more than 200,000 research articles for findings that might be relevant to the treatment of COVID-19.
Service
It can improve some aspects of service delivery:
- NLP tools like Google Translate are making communication easier across the language divide, opening numerous opportunities – personal, business and societal
- Recommender algorithms like those used by streaming platforms can guide us to content that is of interest to us.
Decision-making
It can combine a lot of data into a more digestible form to assist with decision-making:
- Medical diagnoses are crucial to get right. Machine learning systems can help to process a lot of data and/or X-rays to suggest possibilities and out-perform human experts. For example, a 2020 study reported in The Lancet medical journal found that an ML algorithm showed better diagnostic performance in breast cancer detection compared with radiologists.
- Organisations (both government, NGO and corporate) can similarly use machine learning tools to inform their decision-making.
The dark side of machine learning
The data and machine learning revolution also has significant downsides.
Misuse of data
This is the use of data without permission. This is well exemplified by the Cambridge Analytica (CA) scandal where, among other things, CA harvested the personal data of up to 87 million Facebook users through an app called This is Your Digital Life. Users gave the app permission to acquire their data, which in turn gave the app access to their network of Facebook friends. Only about 270,000 users actually used the app, meaning the vast majority of people whose data was taken by CA had not given permission for their data to be used.
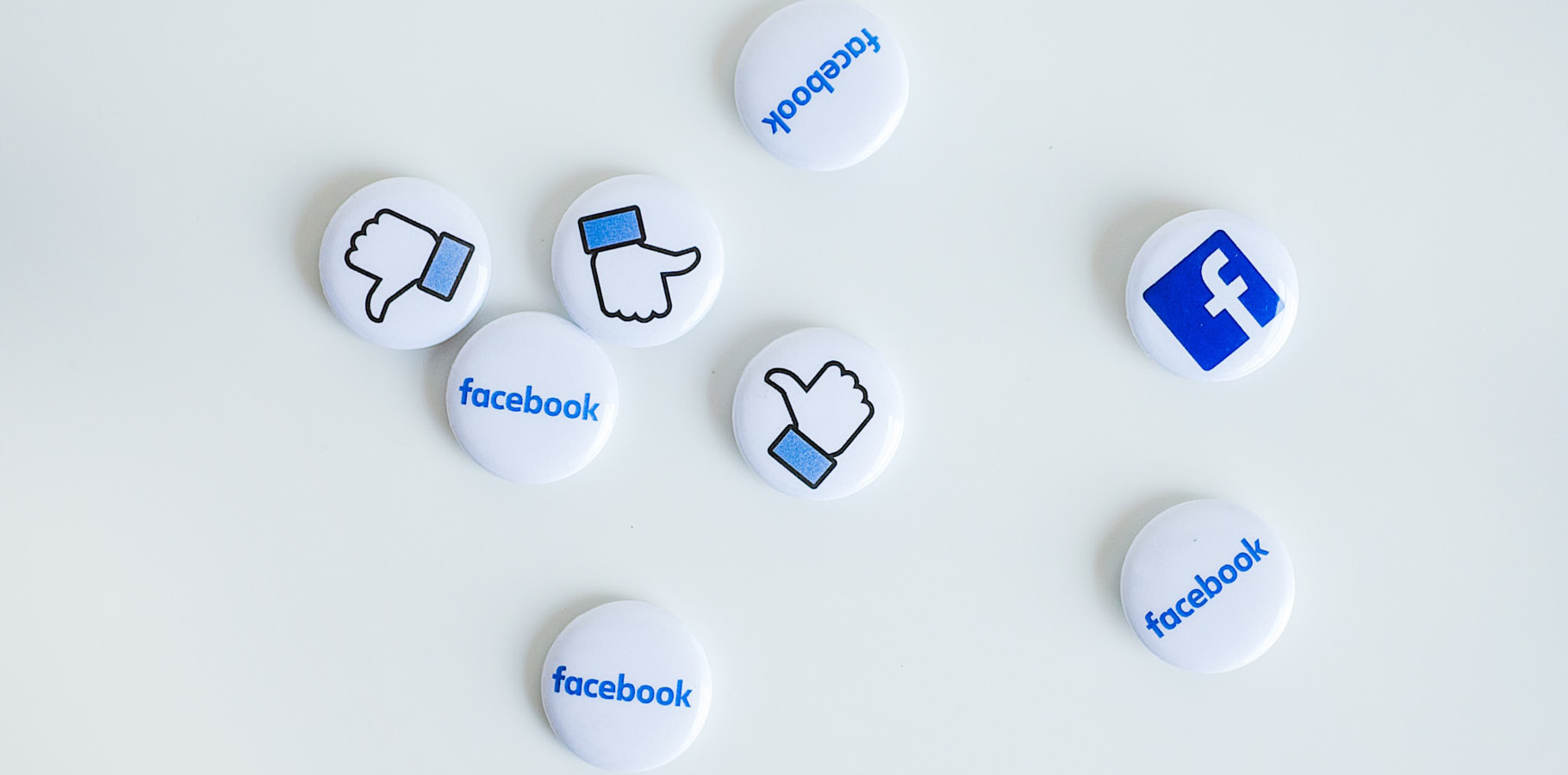
Many journalists and experts consider Facebook is shaking the foundations of democracy
However, the use of data is troublesome even when internet users ‘consent’ to share their data. How many of us actually read the small print outlining which permissions are granted? It’s likely that consent is frequently given without a true understanding of what exactly is being granted. And even if an individual denies permission, the CA case demonstrates that our social network contacts can unwittingly release our data without our explicit permission.
Creation of echo chambers
Platforms like Google and Facebook want us to keep coming back. To ensure this, they show us content they believe we are interested in, rather than a balanced view of whatever issue we are researching, be it climate change or politics, for example.
The polarised political scene in the United States, for example, has highlighted the issue of ethical responsibility for social media giants, especially in considering the broader implications of showing people content that aligns only with a user’s opinions.
Similarly, climate change denial and anti-vaccination campaigns owe no small part to recommender algorithms (such as those used by Google, YouTube and Facebook), sending people down a rabbit hole of anti-science rhetoric. If you want a good illustration of this, watch a YouTube video on something like the Flat Earth conspiracy theory and note the subsequent recommendations you receive.
Externalities/unintended consequences
In economics, an externality is a cost or benefit caused by a producer that is not financially incurred or received by that producer. In the world of machine learning, this could also be rephrased as unintended consequences, a kind of digital or cyber pollution.
It’s unlikely Mark Zuckerberg set out to shake the foundations of democracy when he set up Facebook, and yet many journalists and experts consider Facebook does just that, both through the creation of echo chambers and facilitating the spread of misinformation. Even if corrected or removed afterwards, the misinformation lingers. As the Anglo-Irish satirist Jonathan Swift wrote in 1710, “Falsehood flies, and the truth comes limping after it.” Recent reporting by the New York Times suggests a conflict within Facebook between maximising user engagement and platform growth, and reducing the spread of false or incendiary information.
In her book Weapons of Math Destruction, Cathy O’Neill argues that US university rankings introduced in the 1980s by a single news magazine created a feedback mechanism with self-reinforcing rankings – that is, lower ranked universities would be avoided by top students and academics, funding would reduce, and the ranking would fall even further.
She also makes several other arguments, notably that the omission of college fees from the metrics used to assess universities is one contributor to the high cost of education at prestigious US universities. Dramatically altering the third-level education environment was not the objective of the news magazine, rather it was focused on ensuring its own survival and selling copies.
So what can we do?
There’s an obvious need for a societal response to these challenges. Regulations such as the EU’s General Data Protection Regulation (GDPR) are a small step in the right direction. A set of GDPR provisions are targeted towards AI, restricting automated decision-making (ADM) and profiling when there may be ‘legal’ or ‘similarly significant’ effects on individuals (for example, the right to vote, exercise contractual rights, or effects that influence an individual’s circumstances, behaviour or choices).
The ePrivacy Directive and GDPR mandate that users must be able to deny access to cookies. However, it’s much easier for EU residents to click the Accept All Cookies button once, than have to go through the step of rejecting all cookies and saving this setting each time they visit the website. And that’s assuming the website is available – some content is not viewable within Europe to those who deny cookies.
Clearly more needs to be done. While the systemic nature of the risks of machine learning and big data demand a system-wide approach to its regulation, it’s also important that individual users and developers of machine learning tools think about their own planned use and ensure that it’s ethical.
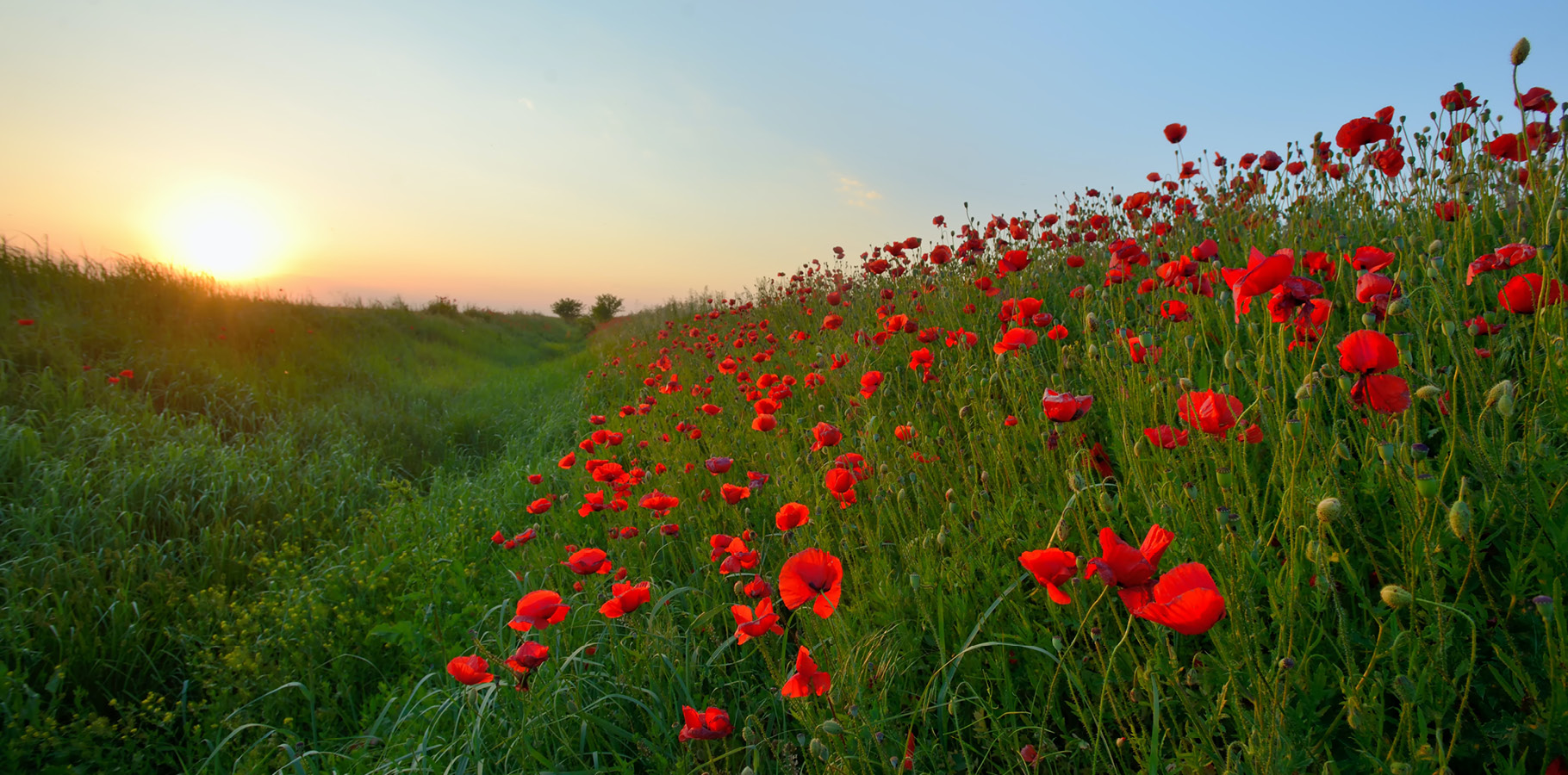
Experts suggest Just War Theory can be applied to AI to achieve greater fairness
What is Just War Theory and what can it teach us?
On the back of the growing concern over the use of machine learning and the broader field of artificial intelligence (AI), Professor Seth Lazar, of the School of Philosophy at the Australian National University, and project leader of the interdisciplinary research project Humanising Machine Intelligence, suggests using Just War Theory as a philosophical framework. Just War Theory is studied by theologians, ethicists, and policy and military leaders. It aims to set out conditions that must be met for a war to be considered just. There are two components:
- Jus ad bellum: the right to go to war. In other words, can this war be justified? For example, a war to remove a genocidal dictator might be considered justified but a war to steal natural resources of a neighbouring country might not. Proportionality is also an important consideration here – the anticipated benefits must be proportional to the expected harms of the war.
- Jus in bello: this considers how combatants should wage war – what acts are justified and what are not. For example, civilian targets would be prohibited, while prisoners of war should not be mistreated. Again, proportionality is important – the harm caused to civilians and civilian infrastructure must not be excessive relative to the expected military gain.
International law, which contains some laws around war and military conduct inspired by Just War Theory, can be used to prosecute those accused of war crimes – for example, the Nuremberg Trials after World War II and the International Criminal Tribunal following the Balkan wars of the 1990s.
Applying the past to the present
Professor Lazar suggests asking similar questions of artificial intelligence:
- Is it justified to use AI in the first place and in what circumstances? (jus ad bellum)
- What is an acceptable way of using AI? (jus in bello)
The second question is discussed a lot in machine learning and wider circles, where concepts of fairness, ethics and bias are regularly considered, but perhaps the first question is not addressed as often as it should be.
Given the huge potential upsides and widespread use of machine learning and big data, a moratorium on its use is very unlikely to happen. Instead, as we grapple with the issues of being a society that is undeniably data driven, we should all ensure that any applications of it under our control are justified and fair.
How can organisations – how can you – be more proactive about fairness?
As business and government leaders look for ways to address issues of ethics and fairness with data and data technology, a good starting point when setting up (or reviewing) a system that uses machine learning is to consider if you should be using machine learning for this task in the first place. It’s a complex question, so answering the following may guide you to a decision:
- What outcomes are you trying to achieve?
- Are there unintended consequences (especially negative) of what you propose?
- Are the potential benefits of the outcomes proportional to the potential harms of the process?
The importance of diversity
For many of these discussions, diversity is key – get a diverse set of stakeholders in the room, ideally including both those using and those who will be affected by the system, and have a robust conversation about all relevant issues.
Part of this conversation should consider what you are currently doing. Ethics and fairness apply whether you use machine learning or not, so review the current state of play and whether your proposal improves this. Even if your proposal isn’t perfect (and it won’t be – we live in an imperfect world), it may be a sufficient improvement over the status quo to justify its use.
The question of proportionality is critical here. Facial recognition software has many known problems, particularly for those with darker skin, but there may be some justification and acceptance for using it as part of a detective toolkit to find a mass murderer rather than a petty thief, and so long as there is appropriate human oversight.
Be vigilant at every stage of the process
If you conclude there is a justification for using machine learning, then you can move onto the second question – how can you use it in an ethical way? It’s important to note that you need to think of the system as a whole – from data collection to modelling to application. You can’t just focus on the machine learning component – a fair model means little if the way in which it’s used is unfair. Problems with a system can occur at any point in the pipeline, and not only at the machine learning stage.
Next week in part two of our ethical machine learning series, we delve into what it means to be fair, the surprising ways bias creeps in and how to ensure dystopian sci-fi scenarios remain in the realm of fantasy.
Related articles
Related articles
More articles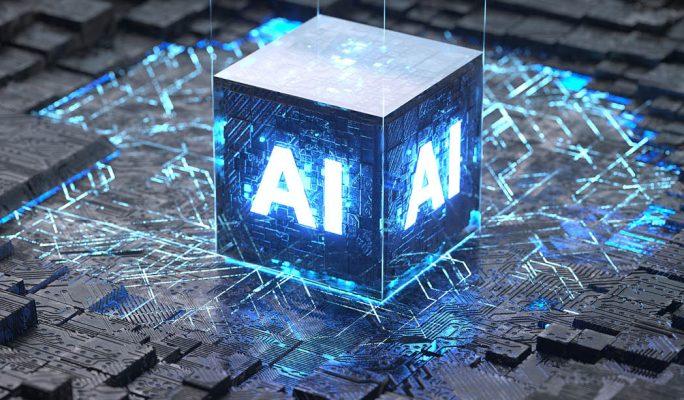
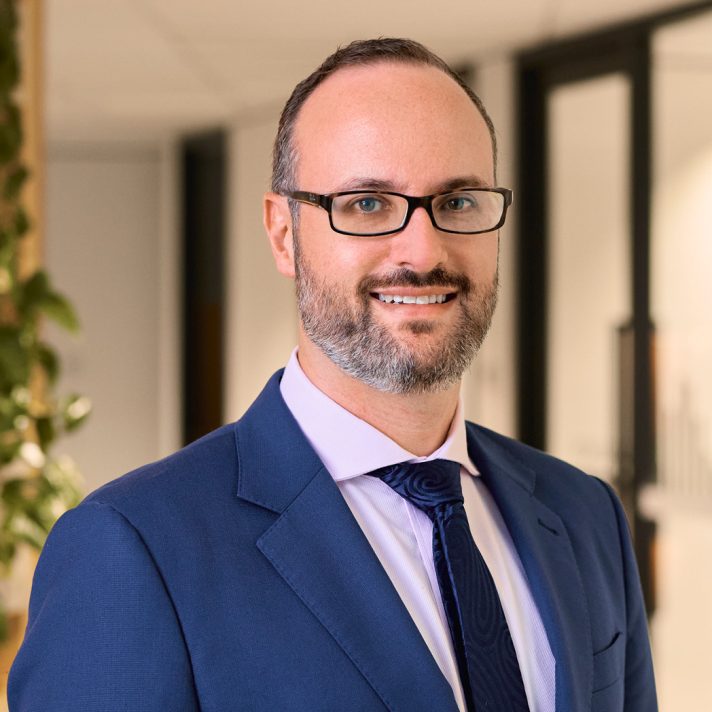
How AI is transforming insurance
We break down where AI is making a difference in insurance, all the biggest developments we’re seeing and what's next for insurers
Read Article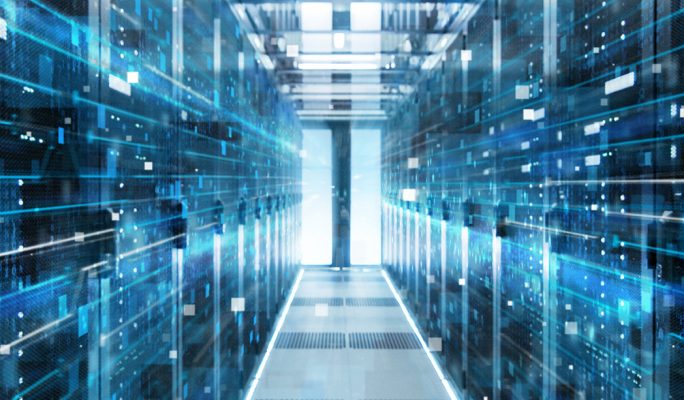
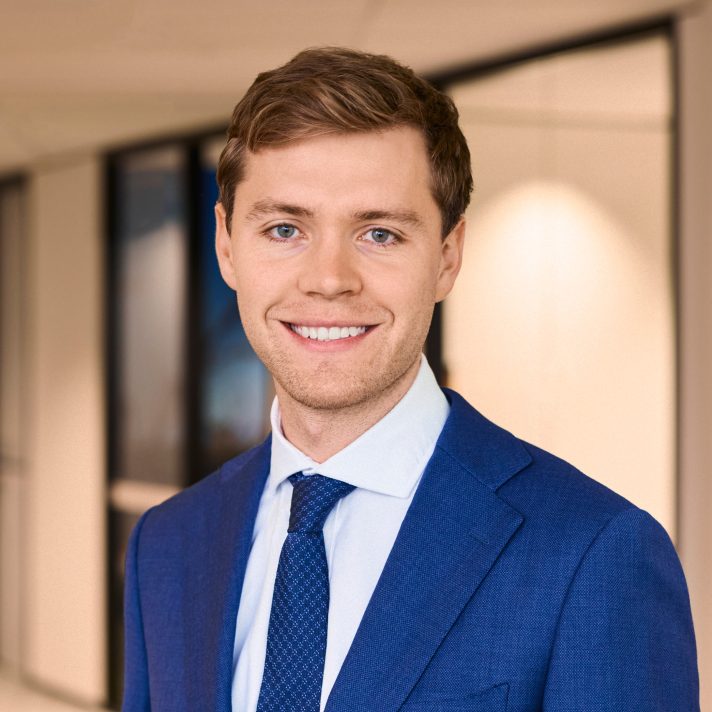
Accuracy and causality the hot topics in data science at SIGKDD 2022
Tom Moulder shares his top picks from the lively discussions at the recent knowledge discovery and data mining conference in Washington DC
Read Article